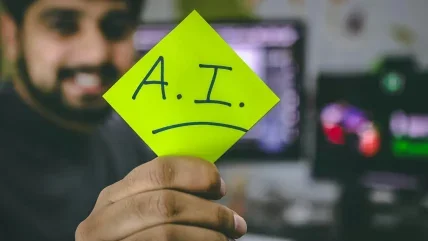
In the international underground infrastructure sector, moves to adopt artificial intelligence (AI) and a start to address data strategy are getting underway. Prof Thomas Marcher, head of Graz University of Technology’s Institute for Rock Mechanics and Tunnelling (RMT), and a partner in Skava Consulting, says advances regarding AI in tunnel applications are being made in a few areas already. But oversight by engineers remains vital.
“The immediate benefit of this technology is improved classification efficiency and self-consistency but the results still need to be critically checked before they are used for decision making,” he says.
Progress includes research toward the eventual development of autonomous methods and systems and equipment capabilities, such as rock mass classification, updating geological information ahead of the tunnel face, grouting, and underground transport systems and logistics. The latter is being pushed by the needs of the mining industry, Marcher tells T&TI.
Switzerland-based consultancy Amberg Engineering is making strides in strategically drawing upon its wealth of historical data to train public algorithms for effective use in the tunnelling sector, as well as gathering new data to work with building information modelling (BIM). Krishna Panthi, Professor of Geological Engineering at the Norwegian University of Science and Technology (NTNU), says researchers have used data from jumbo drilling LiDAR scanners. Eventual 3D coverage of whole tunnels would be a “landmark in our field,” helping stability control, Panthi tells T&TI.
Tunnelling equipment manufacturer Robbins is looking at the potential for greater data collection from more sensors on TBMs, and is currently exploring the use of command chairs for both training and possibly the remotely-based control of machines.
Infrastructure software company Bentley Systems is also drawing upon AI and major use of data for its iTwins system.
Freely-available satellite radar data offers more scope for ground monitoring during tunnelling, a University of Leeds’ spin-out company SatSense tells T&TI. While much is beginning to happen, AI has a long way to go and so the need for tunnellers’ expertise will remain.
NEW TOOLS
AI needs two things – good algorithms and huge quantities of data. The AI algorithms are trained by one of three main ways of ‘learning’:
- Supervised learning (if there are lots of matched datasets).
- Unsupervised learning (far less matching data, if any) or
- Reinforcement learning (very little data at all, initially).
Graz has a research group, established by its RMT institute, to investigate machine learning in geotechnics and tunnelling. According to Marcher and the group, supervised learning can have good datasets from sequential activities, allowing classification of TBM data, interpretation of cone penetration tests (CPT), and detection of damage, and rocks. Research included cooperation with the Brenner Base Tunnel.
At Brenner, on the Ahrental-Pfons Lot, the dataset was built using geological data from the exploratory tunnel to align with recorded measurements on the follow-on main tunnel TBMs.
The TBM parameters included advance speed, rotational speed and torque of the cutterhead, advance pressure, total force, penetration rate, pressure of crown support cylinders, and path of crown-roof support cylinder. Various ratios are recorded, too. Pre-processing of data would adjust for events such as standstills and start-up peaks. The modelling analyses also explored safeguarding against bias in the data. A rock mass classification system resulted, valid only for the stretch of geology and the tunnels.
Unsupervised learning can do clustering for geotechnical data pre-processing, detect anomalous displacements, and perform visualisation. Research has included cooperation with Austrian company Geodata.
Reinforcement learning is the toughest challenge but can come round to learning strategies comparable to real world tunnelling, and – with process optimisation – possibly discover new tunnelling strategies, the research group says. There is also the possibility of advancing toward autonomous drilling systems. Research has been done in cooperation with DSI and the Norwegian Geotechnical Institute (NGI).
Marcher says progress is quickest and easiest with supervised learning but the approach is also the ‘most sensitive’ to risks around bias, and data must be ‘true.’
There are no standardised, reference datasets of different rock types for general use in AI analysis, and it is important therefore to have “meaningful data and avoid training with data in the wrong way,” he says. That means strategy, data strategy.
DATA STRATEGY
Many industries move fast to adopt technology. However, this has happened less so in construction historically, including in tunnelling. But speed leads to a need for strategy.
Data strategy sits where construction meets computer science, or what is termed ‘construction informatics,’ says Dr Ioannis Brilakis, who heads the Construction Information Technology Laboratory at the University of Cambridge.
Brilakis tells T&TI that the linear nature of tunnels is actually an advantage, as there exists “a large degree of repeatability of both the products and the processes.” However, he says the industry is challenged over data in many ways. Generating and cleaning-up data represent costs and what transpires in the supply chain affects how those costs are addressed. “Without sufficient data and integration, it is impossible to leverage AI effectively,” says Brilakis.
A move to lead on digital strategy is part of a new Masters in Digital Engineering Management (DEM) launched in 2020 by University College London’s (UCL) Bartlett School of Construction and Project Management.
Dr Eleni Papadonikolaki, course leader, and Associate Professor in Digital Innovation and Management, tells T&TI: “A data strategy needs to be defined before modern data science methods, such as AI, are implemented.” The DEM MSc looks at digital strategy as a prerequisite to developing AI tools and leveraging the wealth of data in the construction and infrastructure sectors.
Papadonikolaki says: “Data strategy, like any strategy, is related to understanding how an organisation or project will profit and meet business goals from sophisticated use of data.”
At the Norwegian University of Science and Technology (NTNU), Professor of Geological Engineering, Krishna Panthi, agrees that greater focus is needed on data strategy. “The new generation of rock and tunnel engineers must be guided in this direction,” he tells T&TI.
The combination of tunnelling and computer expertise on AI needs to be worked out as more data is going to be generated, he says. “The flood of data is the major concern here, in Norway, too,” he adds. “Like it or not, AI will emerge in one way or another in our industry and will have a greater role in the coming years.” Marcher says data strategy is “a very complex field” around data mining and choosing the most effective algorithms and learning methods. Tunnel engineers applying machine-learning techniques will need support from data scientists with deeper skills in maths and physics, but would also gain benefit from experts in law and psychology, he believes.
DATA SOURCES
Tunnel works offer abundant potential sources of data for AI algorithms. Below ground, much development has been underway with laser and 3D scanning of tunnels in recent years, and detailed surveys, such as Trimble’s systems, were recently used for as-built surveys on part of the Grand Paris metro. ITA’s virtual WTC 2020 conference heard a keynote speech from Tongji University, Shanghai, on adding binocular photogrammetry to 3D scanning for detailed digital model construction of rock features and joints.
For condition monitoring, Amberg developed an AI system trained on its own historical archive of images, T&TI was told by Veronika Petschen, tunnel digitalisation manager. “The AI–machine learning recognises cracks, wet areas and other phenomena on the tunnel surface automatically,” which reduces work time “drastically”. Comparison of inspections over time allows any progression of damage to be easily checked.
Petschen explains that image and object recognition constitute key areas for the company, and she expects them in future “not only to analyse the state of the built environment but to observe construction sites.”
Philipp Dohmen, Amberg’s project leader for digitalisation, explains that TensorFlow has been used to train a neural network for the image recognition work. In addition, object recognition can transfer point-cloud data into BIM objects, and entity extraction algorithms dig into documents and archives. But collecting digital data related to tunnels is also happening above ground – in fact, high above it.
Tim Wright, co-founder of SatSense and a professor of satellite geodesy at Leeds, is in talks for tunnel-related work for its ground movement monitoring algorithms. The system has already ingested freely available satellite data and discovered the ground movement footprint matched to the Northern Line Extension in London, he tells T&TI. “The AI algorithms pick up those signals very clearly,” he says.
SatSense was created in 2018. It uses data based on satellite radar interferometry, including from Europe’s Sentinel-1 satellite which makes four passes of the UK every six days. Wright explains that the abundance of data allows for continuous ground deformation studies through automated production of datasets. SatSense works also with the University of Bristol on developing AI algorithms. Data strategy needs to be a combination of ground-based and satellite measurements, with AI part of the mix, Wright adds. SatSense is part of a project funded by the Centre for Digital Built Britain, at the University of Cambridge, to compare and combine satellite and ground-based monitoring of critical infrastructure. SatSense is also part of the project review group for the Construction Industry Research and Information Association’s (CIRIA) work to produce a report for infrastructure asset owners on satellite remote sensing.
Beyond the spatial aspect, AI is also being used to examine value-based variables that permeate entire projects, such as the carbon and cost estimating tool being trialled on a number of HS2 rail sites by Skanska Costain Strabag JV. Others on the initiative, which links to the BIM models, include Mott MacDonald, software firm Nomitech and the project client.
BIM was also important to Implenia’s recent success in securing the Lysaker-Fornebu rail tunnel and underground station contract in Norway. As BIM works with visualisation systems, on this project Implenia used SiteVision as an augmented reality tool to support concept work at the tender stage, bringing the design into the real environment and reduce somewhat the initial need for scans and aerial drones.
Implenia tells T&TI that AI and data will have increasing influence on projects in future. The contractor also uses AI for its value assurance process, addressing risks across the project’s entire lifecycle.
In discussing big data, Robbins Vice President of Operations, Steve Chorley, explains that it is not unusual for contractors to be on the surface or remotely monitoring operations; for example, last year, 24/7 monitoring occurred from Madrid of a contractor’s drive in Brazil.
Availability of data can also help where training support might be needed. Robbins has worked on a ‘command chair’ technology to provide formal, offsite pre-training for TBM operators to help improve safety and performance. The chair system and screens is self-contained and can be despatched early to site for training. Incorporating data from the live TBM could take this further by enabling real-time training, he adds. The company is also contributing to a TBM simulator being developed by the Colorado School of Mines.
Further developments with algorithms toward the autonomous operation of TBMs have taken place with contractor MMC-Gamuda working with a ‘plug and play’ system on a part of the Klang Valley Metro Line 2 in Kuala Lumpur, Malaysia, and presented at WTC 2020.
PREPARATION
Construction has not been a fast adapter. Marcher says the biggest issue is unfamiliarity, not resistance to AI and data. Young engineers are interested, he says, judging by the successful inclusion of AI elements in Graz’s Masters in rock mechanics and tunnelling, starting two years ago.
“We are transforming from a standardised safety-based design approach towards a data-driven design approach,” Marcher says. He adds that funding is due to be sought from the EU by a group of international organisations, led by Graz and Norway’s NGI, to support education and knowledge transfer across Europe. Simulated projects could be another step in developing skills and abilities.
However, tunnel engineers applying machine-learning techniques would not be starting from scratch. Prior education in maths and statistics have laid the groundwork, and some appreciation of network logic and coding with Python are needed. Along with its MSc, Graz also runs short courses for students and professionals.
NTNU’s Panthi also advocates for the shift in traditional education to accommodate digital engineering, as data-flows rise. Data strategy and AI are coming into teaching on tunnels and geotechnical engineering at NTNU, at postgraduate level.
“It is important that academic institutions, in collaboration with industry, work to train a new generation of engineers to understand and use data correctly,” says Panthi.
CONCLUSION
While the adoption of AI is accelerating fast, the challenge for all will be getting and dealing with the huge quantities of data that are involved. Marcher believes that there is going to be far more research and more adoption of AI in the tunnelling sector over the next 5-10 years.
Dohmen sees the potential for leverage through scaling. “Whoever can turn sub-surface ground engineering know-how into algorithms can support any project in the world,” he says.
The insights and experience of geologists, geotechnical engineers, tunnel designers, builders and others look set to be as important to project success as ever. Arguably more so, to check the AI modelling outputs. Panthi says: “No matter how clever AI is, our control will be equally important to correctly assess the conditions. Complete trust is not possible and subjectivity will always be there. And Marcher adds: “Comparison is important, and so are cross-checks.”