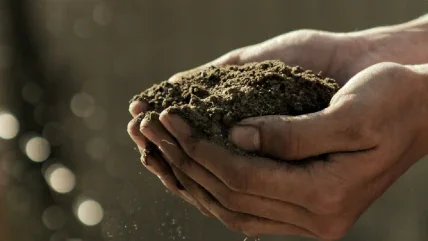
In presenting a methodology for quantitative assessment of soil transitions and critical subsurface features for tunnelling, the online talk to BTSYM discussed a soft ground tunnel project (Anacostia River Tunnel) where geostatistics-based methodologies were used, enabling verification against, and so validation by, actual TBM data.
The research grew out of a strong interest to develop cutting-edge digital solutions that would help with risk management in design workflows, TBM data analytics, visualisation of geotechnical data, and tunnel risk quantification.
Studies and research has brought a PhD in Underground Construction and Tunneling from Colorado from School of Mines, an MS in Geotechnical engineering from Virginia Tech and interesting work with consultants Aecom (a geotechnical and tunnel engineer on Mumbai Metro Line 3, India) and CH2M (several US federal- and state-funded transport projects). Strong involvement in the industry includes being Chair of Underground Construction Association’s (UCA) Young Members’ committee.
MOTIVATION
When considering the problem of quantifying soil transitions, the motivation for the research was to solve the problem for tunnelling contractors faced with the question of soil transition occurrence in the longitudinal and transverse directions along a proposed tunnel alignment. The lack of knowledge of the soil transition zones could result in reduced TBM advance rates, improper regulation of face pressures, creation of sinkholes, and inrush of groundwater.
The lack of sufficient detailed ground awareness can result in severe damage to urban infrastructure, such as in the Kolkata metro project, in India, in 2020.
Another instance – considered in a British Tunnelling Society (2005) report and also research by Clough and Leca (1993) on Washington Metro Area Transit Authority (WMATA) – correlated lack of knowledge of mixed strata with ground deformations.
In Changsha Metro Line 4, in China, about 50mm of surface settlement was observed due to the lag in chamber pressure regulation as the TBM advanced from low to high permeability ground.
From case histories worldwide, it is evident that quantification of the uncertainty of soil transition zones requires more attention to enable efficient tunnelling performance.
In current geotechnical and tunnelling practice, the soil transition location uncertainty is often addressed only qualitatively with a ‘?’ in ground profiles. These are incorporated into Geotechnical Baseline Reports (GBR), which are integral risk allocation documents for tunnel projects, and they are of key utility and significance when seeking to resolve claims over differing site conditions (DSC) and any financial litigation.
Due to deterministic interpretations, such ground profiles within the GBR provide only one, unique, boundary between soil units (SU). The ground profiles fail to include a level of confidence for the given soil transition locations and profiles, and, consequently, do not quantify the associated uncertainty in the longitudinal direction of the tunnel and at the cross sectional area of the TBM face.
To get an appreciation of the large magnitude there can be in DSC claims, in early 2014, Seattle Tunnel Partners, the Design-Build team constructing the Alaska Way Viaduct Tunnel, submitted a DSC claim worth US$20 million to the road tunnel project’s owner, Washington State Department of Transportation, after having completed only about 10% of the TBM drive.
The consequences of risks and impact on the community have skyrocketed with projects in complex environments. There is more at stake now than ever. It is our responsibility, as engineers, to find ways of dealing with uncertainty and build the community’s confidence in tunnelling. There has been previous effort to quantify ground uncertainty but not much work has been done on characterising the subsurface features that are critical to tunnelling.
The research undertaken, therefore, is helping to move towards a more quantitative over qualitative assessments to include in the GBRs and so aid the tunnel contractor with more informed guidance in risk mitigation.
MODELLING SPATIAL VARIABILITY AND UNCERTAINTY
Geotechnical spatial variability is a result of different processes by which soils and rocks are deposited. Depending on the method of deposition, physical and engineering properties will vary in space within the deposit to different degrees. This inherent spatial variability is not completely captured even by rigorous site investigation programmes.
Underground construction and tunnelling work requires tools capable of capturing the variations and uncertainties in material properties. It is also critical to consider the scale at which spatial variability should be modelled.
These spatial variations and uncertainties of geological-geotechnical data can be addressed using geostatistics and random field theory.
These are, generally, two common approaches to estimating the spatial correlation of geotechnical parameters: one uses semi-variance function from geostatistics; the other uses an autocorrelation function from random field theory and time series analysis.
For the semi-variance function, the semivariogram (also called the ‘variogram’) measures the average dissimilarity between two variables – such as between values of a parameter Z separated by a distance h, ie one value of Z at location u and another value at location (u+h). The variogram consists of the nugget (microscale variability and measurement error), sill (global variability in the direction of interest), and correlation length (also known as the range, which is the distance at which the data are no longer spatially correlated).
For geotechnical engineering, and in particular for tunnelling applications, high quality knowledge of ground conditions governs the project procurement, progress, and success. Therefore, as engineers, we are interested in ground models that are geologically realistic, incorporate small-scale variability, and can help evaluate uncertainty from available investigation data.
For this, a stochastic process is adopted – taking a collection of random variables with multiple outcomes, which differ from one another within the set, in terms of properties of modelling techniques, but are considered equiprobable. Geostatistical techniques based on stochastic algorithms are used to estimate the spatial correlation/variability of geotechnical parameters. Applying these to borehole data has increasingly gained interest. This is particularly so for specific tests/ parameters, including standard penetration test (SPT), cone penetration test (CPT), soil stiffness, soil type, rock mass characterisation. What parameters to model is still very much a research question.
The research group at the Colorado School of Mines have, since 2012, applied the results of geotechnical parameter spatial variability to help in various analyses, such as tunnel risk assessment, decision making, and optimising risk mitigation plans.
The general workflow for modelling the soil conditions from borehole data uses the pluri-Gaussian simulation (PGSIM) technique, which is a type of stochastic algorithm. PGSIM is a complete approach as knowledge of a geological depositional environment can be applied towards simulations. The completeness of its approach comes from the ability of the technique to overcome most limitations on simulation methods – mainly of not capturing spatial changes in categories, such as geological unit proportions, contact relationships, and geological realism within realizations.
Each outcome of the stochastic process is called a ‘realization’ and multiple such equiprobable results can be generated, with each one capturing the degree of heterogeneity and spatial variability of the stratigraphic units. The set of realizations differ from one another in terms of properties of modelling techniques but are considered equiprobable under the assumption of reproduction of probability distributions and variograms.
Further, it offers a flexible framework, aiming to reproduce: (1) the contact relationships between units; (2) the unit proportions; (3) spatial correlation structure; and, (4) available conditioning data (i.e. available borehole data).
The PGSIM technique has found application in modelling petroleum reservoirs and also addressing environmental science problems.
GROUND MODELLING: ANACOSTIA RIVER TUNNEL
An example of the probabilistic approach is its application for a research study, in 2021, on the Anacostia River Tunnel (ART) project, in Washington, DC, that is part of DC Water’s Clean Rivers initiative. The probabilistic approach was applied to estimate the soil transition location uncertainty between cohesive (G1/ G2) and cohesionless (G3/G4) soils within the tunnel envelope.
For the geostatistical modelling, a 3D simulation grid was generated that extended out 50m on either side of the ART alignment. Borehole data were discretised (representatively sampled) into the 3D grid cells (also termed ‘voxels’). The dimensions of each cuboid cell, in this case, was 1.8m long to match the tunnel ring length, 1m vertical to match the resolution of vertical sampling in the borehole, and transversely they were assumed to extend 1.8m.
It is recommended that 3D models of ground conditions should be developed to quantify the location uncertainty of soil transitions, conditioned to the borehole data samples and reflecting them having been drilled offset to the tunnel alignment, providing spatial variability – unlike 2D models, which cannot be used to quantify uncertainty.
On the ART project, the PGSIM modelling technique was applied to the borehole data and nearly 500 equiprobable realizations of the ground conditions were generated in terms of soil units. These SUs were simulated, at each grid voxel, by considering their proportion from the borehole data, the probability of transition between SUs, and their spatial variability.
The number of realizations were determined to find confidence in the repeatability of results from PGSIM modelling – meaning the model is maintaining consistency in outputting a soil type at a voxel. SUs with the maximum probability of occurrence (ratio of number of occurrences in all realizations to the total number of realizations) in the voxel is assigned as the most-probable SU. Uncertainty in the SUs was quantified using an information-entropy-based approach, which for a system with a discrete number of probable outcomes (or categorical data outcomes) is a relative measure of ‘missing information’.
SOIL TRANSITION LOCATION UNCERTAINTY – Longitudinal Direction
The quantification of soil transition location uncertainty begins with evaluating the proportion of G1/G2 and G3/G4 soils within the tunnel envelope from multiple realizations, and visualisation of soil proportions and Confidence Interval (CI) bands help to do so.
The results can be interpreted quantitatively, such as ‘at ring #500, the proportion of cohesive soil (for 95% CI) within the tunnel envelope ranges between 20% and 50% of the excavated soil volume.’ The quantitative assessment adds insights on expected ground conditions and therefore helps to characterise the soil transition location uncertainty compared to the qualitative information in the GBR.
With the data, the varying proportions of G1/G2 and G3/G4 at transition locations are used to generate a family of empirical cumulative distribution function (ECDF) curves. These are a stepped function and display the cumulative occurrence probability of a specific soil proportion versus ring#. ECDF curves serve as a robust tool to quantify the probability of occurrence of varying soil proportions within the tunnel envelope. The difference in probabilities from the ECDF curves helps to evaluate the uncertainty in the soil transition locations in terms of CI.
For a ring#, therefore, the ECDF is helping to interpret the probability of occurrence of a specific soil proportion.
P95 observations from the ECDF curves for soil transition 2 indicate that the G3/G4 soil proportion within the tunnel envelope increases from 10% to 50% over a longitudinal distance of approximately 80 rings (or 144m with ring length = 1.8m).
The G3/G4 proportion increases from 30% to 50% over about 50 rings.
The family of ECDF curves for soil transition 2 are relatively more spaced out, indicating a slow and gradual transition from G3/G4 to G1/G2 soil.
It is observed that for 90% CI (P95-P5), the width of the uncertainty band for soil transition 2 is larger due to the lack of boreholes and knowledge of the ground conditions.
For the transverse plane, ECDF curves are generated to quantify the probability of encountering mixed-face conditions for mixed-ground transition plane elevations. As shown in Fig 4, the intersection of the ECDF curve with the elevation of the TBM crown, invert and springline is used to interpret the respective probability of occurrence of mixed-face conditions – which are, here, about 83% and 17%, respectively.
The probabilistic approach offers superior and quantitative insights into the expected ground conditions within the tunnel envelope compared to the deterministic soil profile from the GBR.
Key outcomes of the probabilistic approach include: (a) knowledge of the spatial locations; and, (b) quantification of the probability of varying cohesive/ cohesionless soil proportions within the tunnel envelope. The results aid in quantifying the soil transition location uncertainty expressed in terms of CI.
The geostatistical modelling-based probabilistic approach can be applied during the tunnel project’s procurement stage to estimate the volume of a soil type to be excavated, an important element in planning the management of the excavated material.
SOIL TRANSITION LOCATION UNCERTAINTY – Transverse Direction
ECDF curves for mixed-face conditions quantify the probability of encountering mixed-face conditions and also the probabilistic distribution of the mixed-ground transition plane’s elevations.
For each ring#, the intersection of the ECDF curve with TBM crown, springline, and invert elevation is used to interpret the respective occurrence probability of mixed-face conditions.
The occurrence probability is calculated as the difference in the probabilities of mixed-ground occurring at the respective elevations.
On the ART project, the analysis producing the transverse direction ECDFs for ring #325 and ring #1100 show: at ring #325, the probability of encountering mixed-face conditions between the TBM springline and crown is about 13%, whereas that between the TBM springline and invert is about 80%; at ring #1100, the figures are about 83% and 17%, respectively.
An integrated review of the results from the geostatistics based-methodology provides quantitative insights on the occurrence of mixed-face conditions and the tunnel excavation environment compared to the ground profiles from the GBR.
For example, the results from the probabilistic approach could be interpreted as showing that between ring #200 and ring #300, the probability of occurrence of mixed-face conditions varies between 60% and 90%. The mixed-face conditions are expected to occur between the TBM springline and the invert with the transition plane occurring between 3m and 5m (elevations with respect to TBM crown) for 50% CI.
FIELD VALIDATION USING EPBM OPERATIONAL DATA
In terms of methods for validating results from a probabilistic assessment, for the ART project, the soil transition location uncertainty was examined using the rate of chamber pressure dissipation using the operation data collected from the earth pressure balanced machine (EPBM).
The analysis was informed by the Bezuijen and Dias (2017) model that discusses reduction in chamber pressure during standstill, influenced by the hydraulic conductivity of the soil immediately in front of the cutterhead.
Rate of chamber pressure dissipation was examined for each ring to infer the permeability of soil type at the face and compared to the permeability baseline values in a GBR.
From the ART project’s GBR, the baseline permeability of G1/G2 soils was kc ε [1E-9 to 1E-6] m/s, and the permeability of G3/G4 soils was ks ε [1E-6 to 1E-3] m/s. For each ring, the analytical fit to chamber pressure dissipation was used to evaluate the permeability and identify the presence of dominant soil type at the tunnel face.
Figure 6 shows the maximum EPBM chamber pressure dissipation rate between ring #250 and ring# 274 is between 0 and 0.05 kPa/min, except at ring# 273. Low rate of chamber pressure dissipation indicates low permeable G1/G2 soil at the EPBM face. Given no change in the overburden and geostatic groundwater conditions, the observed increase in chamber pressure dissipation rate is very likely a result of formation soil permeability increase at the face.
The observations reveal that the transition from cohesive to cohesionless soil, within the tunnel envelope, does not occur at or near ring #260, as indicated would be the case in the GBR. Results confirm that the transition from G1/G2 to G3/G4 soil occurs (P95 for a 50% or greater proportion of G3/G4 soil at the EPBM face) at ring #275.
Chamber pressure dissipation between rings #500 and #550 was relatively high, between 0.05 and 0.3 kPa/ min, indicating dominant presence of highly permeable G3/G4 soil at the EPBM face. The dip in chamber pressure dissipation beyond ring #550 indicates the presence of G1/G2 soil in relatively higher proportions at the face.
The probabilistic approach shows that the transition from G3/G4 to G1/G2 soil (P95 for 50% or greater proportion of cohesive soil at the face) occurs at about ring #550, compared to ring #520 indicated in the GBR.
VALIDATION WITH MODELS BUILD ON AI
EPBMs are outfitted with extensive sensors to measure both human operations and machine reactions. Measurements are taken frequently (every 5-10 seconds) and are readily available to all stakeholders. Since the behaviour of the EPBM is influenced by the ground condition, it is possible to develop a data-driven model to relate the EPBM construction data with the asencountered ground condition. Such modelling can yield a more detailed and accurate description of the ground to enhance the operator’s geological awareness.
Characterising the as-encountered ground using EPBM data involves finding features as model inputs to compute the expected ground condition as the model output.
The choice of the input predictors, ideally, capture the differences of various ground in aspects such as strength, density, elasticity, etc. Using our tunneling domain knowledge, the following measurements are included: the total thrust force (F) and advance rate (AR), cutterhead rotation speed (ω) and torque (T), chamber pressure at springline (pc), screw conveyor rotation speed (ωs) and torque (Ts), specific energy of excavation (SEE) and field penetration index (FPI), as well as the excavated muck mass (EMM) calculated from the belt conveyor scale.
Except for the EMM, all these predictors are evolving during excavation and are measured by the EPBM every five seconds. To make them a predictor vector of equal length, their average values are taken during stable EPBM advancement. This treatment leads to a predictor vector xi for each ring i, namely xi = [F, AR, ω, T, pc, ωs, T, SEE, FPI, EMM].
Yu and Mooney (2021) utilised EPBM operation and reaction data while tunnelling to characterise the asencountered ground type. A semi-supervised learning (SSL) model – a type of machine learning – is applied. The SSL model uses a small portion of labelled data and a large portion on unlabelled data to interpret the occurrence probability of soils at each ring.
To characterise the as-encountered ground conditions within the ART project, soil fractions within the tunnel envelope were extracted from 43 boreholes by associating the boreholes to the nearest ring. Within the SSL model, each ring was represented by the EPBM operation parameters and the estimated soil fractions within the tunnel envelope for the SSL model training. The data-driven models thus utilised the ring-level EPBM data rather than only the sparse boreholes for prediction. Therefore, the results from the SSL model can be considered reliable to validate the occurrence of transitions at a finer resolution.
CONCLUSIONS
The field validation using EPBM operation data demonstrates the capability of geostatistical modelling in identifying soil transitions.
The geostatistics-based probabilistic approach provides improved understanding and a quantifiable characterisation of soil transition locations over the qualitative interpretations from the GBR.
Such geostatistics-based probabilistic methodologies can be applied during many stages – planning, tendering, construction, and post-construction to help analyse disputes and claims.
The flexibility of applying geostatistical techniques at multiple stages allows for effective risk management and decision-making for contractors, designers, and owners. Although, geostatistics is a powerful tool for investigating the influence of ground uncertainties, it is essential to remember that the results derived from geostatistics are based on the given input of limited geotechnical data.